Description
SAR Image Fusion
Abstract
Unlike multispectral (MSI) and panchromatic (PAN) images, generally, the spatial resolution of hyperspectral images (HSI) is limited, due to sensor limitations. In many applications, HSI with a high spectral as well as the spatial resolution is required. In this paper, a new method for spatial resolution enhancement of an HSI using spectral unfixing and sparse coding (SUSC) is introduced. The proposed method fuses high spectral resolution features from the HSI with high spatial resolution features from an MSI of the same scene. End members are extracted from the HIS by spectral unmixing, and the exact location of the end members is obtained from the MSI. This fusion process by using spectral unmixing is formulated as an ill-posed inverse problem which requires a regularization term in order to convert it into a well-posed inverse problem. As a regularize, we employ sparse coding(SC), for which a dictionary is constructed using high spatial resolution MSI or PAN images from unrelated scenes. The proposed algorithm is applied to real Hyperion and ROSIS datasets. Compared with other state-of-the-art algorithms based on pan sharpening, spectral unmixing, and SC methods, the proposed method is shown to significantly increase the spatial resolution while preserving the spectral content of the HSI. for this we are using the firefly algorithm.
Keywords: Firefly algorithm, robust feature extraction, vegetation images, shadow images, multispectral images
Introduction
Image fusion is a process, which creates a new image representing combined information composed of two or more source images. Generally, one aims to preserve as much source information as possible in the fused image with the expectation that performance with the fused image will be better than, or at least as good as, performance with the source images. Image fusion is only an introductory stage to another task, e.g. human monitoring and classification. Therefore, the performance of the fusion algorithm must be measured in terms of improvement or image quality. Several authors describe different spatial and spectral quality analysis techniques of the fused images. Some of them enable subjective, the others objective, numerical definition of spatial or spectral quality of the fused data. The evaluation of the spatial quality of the pan-sharpened images is equally important since the goal is to retain the high spatial resolution of the PAN image. A survey of the pansharpening literature revealed there were very few papers that evaluated the spatial quality of the pan-sharpened imagery. Consequently, there are very few spatial quality metrics found in the literature. However, the jury is still out on the benefits of a fused image compared to its original images. There is also a lack of measures for assessing the objective quality of the spatial resolution of the fusion methods. Therefore, objective quality of the spatial resolution assessment for fusion images is required. Therefore, this study presented a new approach to assessing the spatial quality of a fused image based on the High pass Division Index (HPDI). In addition, many spectral quality metrics, compare the properties of fused images and their ability to preserve the similarity with respect to the original MS image while incorporating the spatial resolution of the PAN.
EXISTING METHOD
- Averaging and Maximization methods based on spatial level fusion
- Thresholding and K mean clustering methods for segmentation:
- Wavelet Transform
Drawbacks
- Contrast information loss due to averaging method
- Maximization method sensitive to sensor noise and high spatial distortion
- K means – It is not suitable for all lighting conditions of images
- Difficult to measure the cluster quality
PROPOSED METHOD
- Dual-Level Wavelet and Log Ration Transform
- Detection of Backscattering Changes at Building Scale
- Building Detection using NN classifier.
Block Diagram
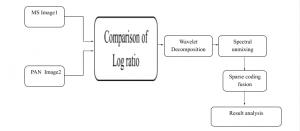
Advantages
- Accurate detection of foreground changes by fusion
- Less sensitive to noises
Applications
- Earth land changes detection in the Satellite field
- Medical field.
Conclusion
Finally, using this hearth fly algorithm we will get the precise area of the building. For this purpose take input images, multispectral images, and dynamic images. Using this we are becoming a fusion image. Then getting different outputs after fusion. That won’t get the precise area of the buildings. For that, we are using robust feature extraction, measurement areas, and shadow of the pictures, vegetation images, detection process, and building masking. And that we are saying pan images are nothing but the fusion-based thing. To detect and locate changes between SAR images from the satellite we are using this project. During this project, we will expect good results by using the firefly algorithm. For that purpose, we will approach these techniques.
SAR Image Fusion
References
[1] S. Dasgupta, C. F. Stevens, and S. Navlakha, A neural algorithm for a fundamental computing problem, Science, vol. 358, no. 6364, pp.793-796, 2017.
[2] X. Tang, L. Jiao, and W. J. Emery, SAR Image Content Retrieval Based on Fuzzy Similarity and Relevance Feedback, IEEE Journal of selected topics in Applied Earth Observations and Remote Sensing, vol. 10, no. 5,pp. 1824-1842, 2017.
[3] L. Jiao, X. Tang, B. Hou, et al., SAR images retrieval based on semantic classification and region-based similarity measure for earth observation, IEEE Journal of Selected Topics in Applied EarthObservations and Remote Sensing, vol. 8, no. 8, pp. 3876-3891, 2017.
[4] X. Tang and L. Jiao, Fusion Similarity-Based Reranking for SARImage Retrieval, IEEE Geoscience, and Remote Sensing Letters, vol. 14, no. 2, pp. 242-246, 2017.
[5] M. Datcu, H. Daschiel, A. Pelizzari, et al., Information mining in remote sensing image archives: System concepts, IEEE Transactions onGeo-Science, and Remote Sensing, vol. 41, no. 12, pp. 2923-2936, 2017.
Customer Reviews
There are no reviews yet.